by Catherine Graham, Johns Hopkins University
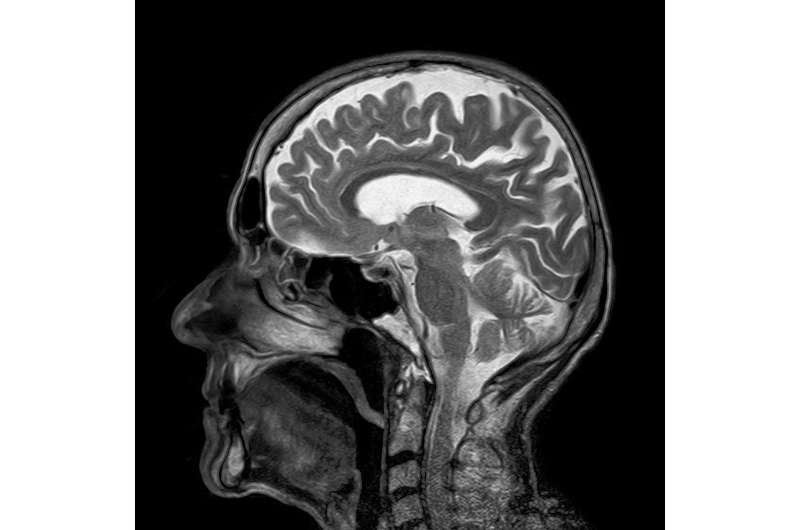
Credit: CC0 Public Domain
Magnetic resonance imaging (MRI) uses magnetic fields to create images of the body that allow doctors to diagnose injury or illness more accurately. Susceptibility tensor imaging (STI), a specialized MRI technique, measures the magnetic susceptibility of different tissues in the brain by quantifying how they become magnetized when exposed to the MRI scanner’s magnetic field. Researchers and physicians can use such information to better understand, diagnose, and monitor neurological diseases such as multiple sclerosis (MS) and Alzheimer’s disease.
Johns Hopkins researchers have recently published papers describing a new algorithm, DeepSTI, that takes data from multiple individual scans and provides a “super-scan” of the brain that includes precise brain tissue susceptibility information. Their method requires fewer images taken in fewer positions compared to traditional STI, making the process faster and more pleasant for patients.
“Usually, STI imaging requires at least six different scans at different head orientations to achieve a good reconstruction, and that’s mainly why it’s not currently broadly used despite its potential to understand the human brain,” said senior author Jeremias Sulam, an assistant professor of biomedical engineering. “Our AI-assisted reconstructions greatly expand the amount of useful information that can be gleaned while requiring much less data, and we hope that will help move this imaging technique from lab to clinic.”
The team’s results appears in the proceedings of the 2023 International Workshop on Machine Learning in Clinical Neuroimaging.
Zhenghan Fang, a biomedical engineering graduate student, is the lead author of the papers. Peter van Zijl, Xu Li, and Hyeong-Geol Shin from the Kennedy Krieger Institute also contributed to the studies.
STI imaging excels in uncovering neurodegenerative processes that affect specific structures in the brain, such as the myelin sheath that surrounds axons and plays a central role in the transmission of information in the brain.
A new algorithm called DeepSTI, developed at Johns Hopkins, achieves a highly detailed 3D-map that shows magnetic susceptibility of tissues in the human brain. Credit: Johns Hopkins University
The team’s new algorithm takes data from MRI scans and generates a high-resolution 3D map of magnetic susceptibility in the brain. Its key advance, the researchers say, is its ability to measure brain tissue sources like myelin and iron using fewer scans than previously needed. Capturing changes in these tissues can help characterize neurological disease type, stage, or progression; for example, their algorithm generated a reconstruction to visualize myelin changes in patients with MS, using data collected from a scan from only one head orientation.
The team recognized the challenges of developing an algorithm to construct an image of something as complex and varying as the human brain, especially with limited information, said Fang. To take an example, imagine being asked to paint an image of a dog but weren’t given information such as the animal’s color, size, or breed; there would be a vast number of solutions to the problem, so you’d need to reduce the number of options and narrow it down to one.
DeepSTI uses machine learning with an approach called regularization that narrows down the number of possible solutions to focus on the most accurate ones. The researchers designed special regularizers using prior scan data to teach the model what good brain reconstructions should look like. These data-driven regularizers steer the model toward the most plausible solution for each new set of scans.
“The algorithm is very good at ‘learning’ what brain images should look like. When we run the algorithm on raw data, it uses those found parameters to reconstruct a clearer and more comprehensive image of the brain,” said Fang.
Eventually, Sulam says, the hope is that their machine learning algorithm will make STI a more accessible imaging option for clinicians and radiologists by cutting down the time it takes to perform a scan and boosting the quality of the image. For now, the team plans to explore how the algorithm could address other difficult science and engineering problems. And the team is especially encouraged by what Sulam calls the “very beautiful” math powering the algorithm.
“Our team is excited about the mathematical framework that underpins the results here. We’ve been able to answer hard questions that we couldn’t answer before, like what are the implicit priors of data captured by these reconstruction algorithms, why this works, and why we should be using this,” said Sulam. These new mathematical results are currently under review and available as a pre-print on arXiv.
The team has made its data, models, and code available as an open-source project for other researchers. “This is a true research success story because not only could it lead to new technologies to aid decision-making in the clinic, but also is opening up new research avenues in more general restoration and reconstruction algorithms and applied mathematics,” said Sulam.
More information: Zhenghan Fang et al, WaveSep: A Flexible Wavelet-Based Approach for Source Separation in Susceptibility Imaging, Machine Learning in Clinical Neuroimaging (2023). DOI: 10.1007/978-3-031-44858-4_6
Journal information: arXiv
Provided by Johns Hopkins University
Leave a Reply