Thought leaders, patient advocates develop guidelines for ethical use of AI in medicine.
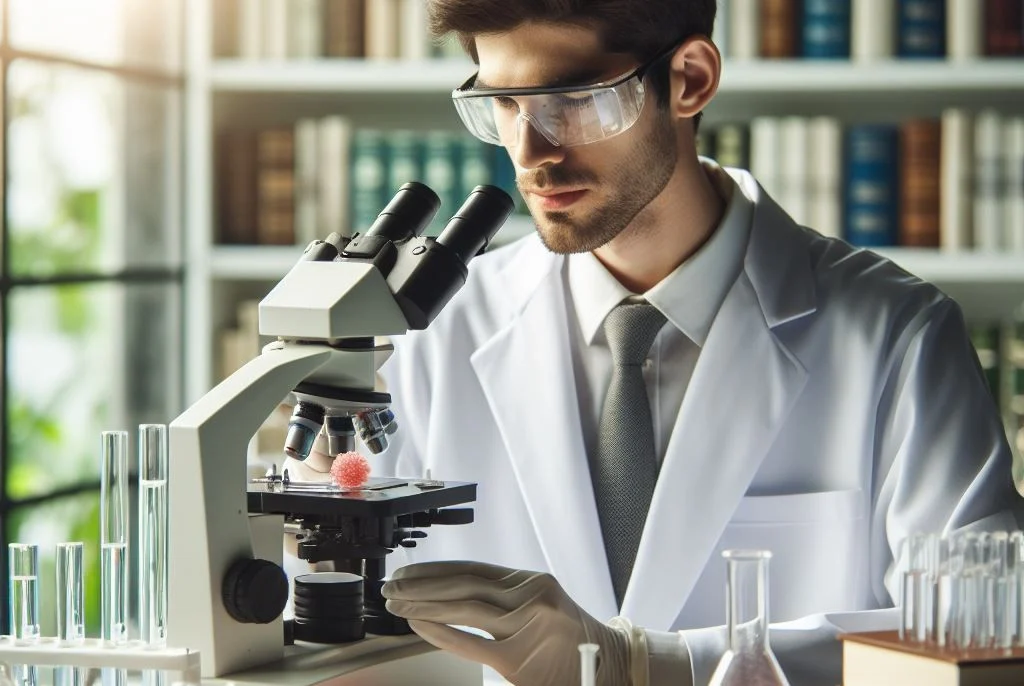
Medical research – artistic interpretation.
From the invention of the wheel to the advent of the printing press to the splitting of the atom, history is replete with cautionary tales of new technologies emerging before humanity was ready to cope with them.
For Zak Kohane, the chair of the Department of Biomedical Informatics in the Blavatnik Institute at Harvard Medical School, the arrival in fall 2022 of generative artificial intelligence tools like ChatGPT was one such moment.
“After going through the stages of grief, from denial to acceptance, I realized we’re on the verge of a major change,” Kohane said. “It was urgent to have a public discussion.”
In academic circles, Kohane has been long known as an AI evangelist. He has studied AI and written about its tremendous promise to change medicine for the better by doing everything from detecting novel disease syndromes, minimizing rote work, reducing medical errors, reducing clinician burnout, and empowering clinical decision-making, all of which would converge to improve patient health.
So why was the news of ChatGPT’s arrival so unsettling?
“It is a mind-blowing technology, yet for now we cannot guarantee that its advice is reliably trustworthy every time,” Kohane said. “Despite their promise, ChatGPT and tools like it are immature and evolving so we need to figure out how to trust their abilities but verify their output.”
For Kohane and like-minded colleagues, one question looms larger than others: How to prevent harm without extinguishing the enormous potential of a promising technology?
With that urgent question in mind, Kohane convened colleagues from across the world, across disciplines and across industries to ponder critical questions about AI in health care. The aim: To develop an ethical framework that would inform and guide policymakers and regulators.
“We have a societal obligation to develop a pathway to guide us in what is a deeply confusing situation,” Kohane told attendees.
During the last two days of October, experts in policy, patient advocacy, healthcare economy, AI, bioethics, and medicine pondered and debated several questions related to the safe and ethical use of artificial intelligence in medicine.
The deliberations culminated in a set of broad guiding principles published simultaneously in Nature Medicine and The New England Journal of Medicine AI, of which Kohane is editor-in-chief. These principles, the participants said, should help inform both the public discussion and eventual regulations of AI in medicine.
The overarching consensus converged on the theme of doing good while minimizing harm. Adopting medical AI will pose challenges, participants agreed, but failure to do so may pose a greater risk, especially where AI stands to yield the greatest benefits, such as in absorbing administrative rote tasks, lessening clinician stress, improving access to care, and reducing medical errors.
Who should medical AI serve?
How should regulators balance the overlapping, and sometimes diverging, interests of patients, clinicians, and institutions in the design and deployment of medical AI models?
Because of the potential for misalignment of incentives and interests, regulation should recognize the heterogeneity of interests and contexts and maximize equity of access.
Panelists agreed that medical AI models should be designed and deployed under the moral imperative to not merely to avoid harm but to do good and achieve maximal benefit for the greatest number of patients.
“Patients should be viewed as the ultimate stakeholders and primary beneficiaries of medical AI,” said Tania Simoncelli, vice president, Science in Society at the Chan Zuckerberg Initiative.
And patients should be actively involved, panelists said.
“Patients need to be active participants in the process of designing, deploying, and using AI, not just the passive beneficiaries of the things that smart people do for them,” said patient advocate and activist Dave deBronkart, known as e-Patient Dave.
Is AI an equal party in the patient-clinician relationship?
AI is not an equal partner in the clinician-patient relationship, attendees agreed, but it can be a useful clinical aid. If so, what liabilities and responsibilities emerge in the doctor-patient relationship when it’s augmented by AI?
Who controls the incorporation of patient data into the training of AI tools?
AI models are only as good as the quality of the patient data they are trained on. Thus, inclusion of diverse populations and a broad range of parameters is essential. Collection of such data during clinical care should rely on opt-out rather than opt-in setups — the latter could exacerbate data disparities because of historical mistrust among some populations toward medical science.
Patients should have the legal right to opt out of having their data used in AI training,
the right to correct errors, and right to be forgotten by expunging their information if they so wish. Finally, consent must be specific, not generic. But opting out may come at the cost of bias, some participants warned. If self-selecting populations choose to have their information included in the dataset, that data may be skewed. So, there should be efforts to ensure that patients make informed decisions about the tradeoffs of opting out. This raises the question of how to make consent informative and thorough, yet clear and easily understood by users.
“Patients are the end users, but many times they do not know that AI is being used on them,” said Maya Rockeymoore Cummings, a non-resident senior fellow at Brookings Metro and a strategic adviser to the Light Collective, a coalition that represents the collective rights, interests, and voices of patient communities in healthcare technology.
“There should be no aggregation without representation — patients need to be involved at every level of the enterprise,” she added.
Any ethical and legal framework, the panelists said, would need to reflect changing cultures and international borders, and the guidance would have to be reevaluated regularly.
Should consumers have access to medical advice from AI?
The real question, panelists agreed, is not whether patients should have access to AI — they should and already do — but how to ensure that AI models provide solid advice and that patients still turn to trusted clinicians to vet AI-generated information.
Educating and empowering patients about the capabilities and limitations of AI should be paramount. Patients should be taught how to ask the right question and give the AI model the right prompt to get reliable answers.
Patients should be reminded of the value of obtaining information from a variety of sources, with AI as a starting point. Additionally, medical AI should divulge the possibility of bias and error based on how it was trained. Such information should be disclosed to users.
Likewise, AI developers need to understand how patients interact with AI and allow users to provide direct feedback after deployment. Testing of the model is important but so is regular validation to ensure it continues to perform with high fidelity over time.
Who pays for AI?
Who pays for the development and ongoing maintenance of AI will have ramifications on how AI models are adopted and used. How can we ensure AI tools are health-focused rather than profit-driven?
Regulation should create performance-based incentives by mandating that private and public insurers reimburse AI use for good patient outcomes.
Regulation should also eliminate perverse incentives by ensuring that the use of AI does not directly increase vendors’ and providers’ revenues. For example, if a company stands to make money from its AI model that improves stroke detection because this would also generate more use of its stroke-treatment devices, this should be treated as a conflict of interest. Transparency and accountability should be required from companies that promote AI tool usage and purchase.
“Could market-testing and economic benefits assessment be part of clinical evaluation of algorithms?” asked Peter Lee, corporate vice president for research and incubations at Microsoft.
As an ethical imperative, AI policies should be focused on the public good, the panelists agreed. Just like drug-makers offer medications for free or at a lower cost to low-income countries, AI tech companies should make a commitment to develop AI tools for free or at a lower cost underserved populations and areas.
David Cutler, the Otto Eckstein Professor of Applied Economics at Harvard, proposed four criteria to guide the evaluation of AI tools: better experience for the patient, better health outcomes, lower healthcare costs, improved workforce wellness as an antidote to clinician burnout.
On the regulatory front, Cutler said, policymakers would need to think about how AI use gets reimbursed. In a fee-for-service model, each time AI is used in a medical office – a radiologist using AI to give a CT scan a second read, for example – the hospital gets paid, either by private insurance or by government payor. In a bundled payment model, AI use is reimbursed generally but not for episodic use. Under the fee-for-service model, a radiologist would be encouraged to use AI with all patient scans to get paid at a higher rate. Fee-for-service, Cutler said, could lead to overuse and create a perverse incentive. Solution: Pay for value to incentivize quality.
In his closing keynote address, Lee underscored the importance of educating users — clinicians, patients, and the public — on what generative AI can and cannot do.
Neural networks have limitations similar to those of the human brain in certain tasks such as performing complex calculations or rote memorization of long texts, Lee said. However, neural networks are exceedingly good at pattern detection, review, synthesis, and critique. This makes them useful tools to help challenge our thinking and in push us to consider different approaches.
“The biggest mistake we could commit is to use generative AI in health care as a computer,” Lee said. “The implicit assumption that this is just another computer system is dangerous.”
Regulation should be narrowly tailored and specific to the various capabilities of AI, Lee added. For example, back-office administrative functions such as writing justifications for physician referrals or insurance coverage for a medication are very different from using AI a diagnostic aid or treatment choice support. These uses demand different guidelines.
Generative AI models are already undergoing refinements that will enhance their ability to access external tools and databases. This should lead to reduction in errors and dramatically amplify their capacity. Access to large, potentially infinite contexts will enable AI tools’ ability for deep personalization. For example, AI models will increasingly be able to remember past encounters with the user and use prior context to personalize output. Another near-future development would be the emergence of autonomous agents that would allow one model to supervise another and spot errors. Thus, Lee cautioned, any regulatory guidelines should try to anticipate this future by factoring in such emerging capabilities.
In a wrap-up lecture, panelist Laura Adams, senior advisor at the National Academy of Medicine, discussed the imperative of governing the hope, hype, promise, and peril of medical AI. She noted that if done right, AI can dramatically change medicine for the better but cautioned that the path to realizing this vision remains uncertain.
Medical AI remains uncharted territory with many perilous turns ahead, including perpetuation of bias, threats to privacy, and a widening of the equity divide, if not properly stewarded.
The gravest threat of all, Adams said, might be failing to govern AI and harness it in service of humanity. To that end, those designing the future of AI-enabled healthcare should proceed with intentionality, respect for the patient, and the humility to realize that this is an “all-teach, all-learn” moment.
In the end, Adams said, AI can offer information but not wisdom, for it is neither sentient nor conscious. It cannot replace the human connection.
“Truly personalized care is about patients feeling seen and heard and having a sense of belonging.”
Source: HMS
Leave a Reply