Medscape Medical News
Richard Mark Kirkner
August 08, 2024
Emerging diagnostic technology that uses artificial intelligence (AI) has demonstrated the potential to free dermatologists from the burden of triaging skin lesions, but dermatology lags behind some other specialties in harnessing the power of AI, and confusion surrounds dermatologists’ role in using this technology, according to researchers and dermatologists investigating AI.
While some AI-integrated devices designed to triage skin lesions have emerged, including one that received US Food and Drug Administration (FDA) clearance earlier this year, it may be some time before AI has a meaningful clinical impact in dermatology, and specifically, for the diagnosis of skin cancer, Ivy Lee, MD, a dermatologist in Pasadena, California, and chair of the American Academy of Dermatology’s augmented intelligence committee, told Medscape Medical News.
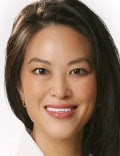
Ivy Lee, MD
“It hasn’t really translated into clinical practice yet,” Lee said of AI in dermatology. “There have been significant advances in terms of the technical possibility and feasibility of these tools, but the translation and integration of AI into actual clinical workflows to benefit patients beyond academic research studies has been limited.” More studies and more “easily accessible and digestible information” are needed to evaluate AI tools in dermatologic practice, she said.
“In dermatology, we’re on a cusp with AI,” said Rebecca Hartman, MD, MPH, chief of dermatology at the VA Boston Healthcare System and director of melanoma epidemiology at Brigham and Women’s Hospital, Boston. “I think it’s going to come and change what we do,” which is especially true for any image-based specialty,” including radiology and pathology, in addition to dermatology, she said. Hartman led a study of one of these emerging technologies, the handheld elastic scattering spectroscopy device DermaSensor, which was cleared by the FDA in January for evaluating skin lesions suggestive of skin cancer.
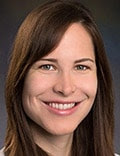
Rebecca Hartman, MD, MPH
Early AI Devices for Skin Cancer DetectionAt the American Society for Laser Medicine and Surgery (ASLMS) meeting in April, a panel explored a number of algorithms with dermatologic applications that use AI to triage skin lesions, including DermaSensor. Others included:Raman spectroscopy, which contains a handheld Raman probe, a diode laser, and a detecting spectrograph. A laser beam — which at 1.56 W/cm2 is below the maximum permissible exposure — focuses on the skin target with a 3.5-mm spot, gathers data on the target, and feeds it back into the unit that houses the algorithm that evaluates the spot analysis. It’s still in the investigative phase. A clinical trial, published almost 5 years ago, demonstrated a sensitivity of 90%-99% and a specificity of 24%-66% for skin cancer.A dermatoscope called Sklip clips onto a smartphone and performs what company cofounder Alexander Witkowski, MD, PhD, described as an “optical painless virtual biopsy” for at-home use. The device uploads the captured image to an AI platform for analysis. It received FDA breakthrough device designation in 2022. At the ASLMS meeting, Witkowski said that clinical performance showed the device had a 97% sensitivity and 30% specificity for skin cancer.DermaSensor, described in the study conducted by Hartman and others as a noninvasive, point-and-click spectrometer, is a wireless handheld piece that weighs about 10 ounces. The unit captures five recordings to generate a spectral reading, which an algorithm in the software unit analyzes. The study found a sensitivity of 95.5% and specificity of 32.5% for melanoma detection with the device.The target market for DermaSensor is primary care physicians, and, according to the FDA announcement in January, it is indicated for evaluating skin lesions “suggestive” of melanoma, basal cell carcinoma (BCC), and/or squamous cell carcinoma (SCC) in patients aged 40 and older to “assist healthcare providers in determining whether to refer a patient to a dermatologist.”
So Many Cases, So Few Dermatologists
In dermatology, AI devices have the potential to streamline the crushing burden of diagnosing skin cancer, said Yun Liu, PhD, a senior staff scientist at Google Research, Mountain View, California, who’s worked on developing machine learning tools in dermatology among other medical fields. “Many people cannot access dermatology expertise when they most need it, ie, without waiting a long time. This causes substantial morbidity for patients,” Liu told Medscape Medical News.
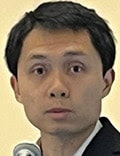
Yun Liu, PhD
His own research of an AI-based tool to help primary care physicians and nurse practitioners in teledermatology practices diagnose skin conditions documented the shortage of dermatologists to triage lesions, including a finding that only about one-quarter of skin conditions are seen by a specialist and that nonspecialists play a pivotal role in the management of skin lesions.
The Centers for Disease Control and Prevention reports that about 6.1 million adults are treated for BCC and SCCs each year. The American Medical Association estimates that 13,200 active dermatologists practice in the United States.
Overcoming Barriers to AI in Dermatology
Before AI makes significant inroads in dermatology, clinicians need to see more verifiable data, said Roxana Daneshjou, MD, PhD, assistant professor of biomedical data science and dermatology at Stanford University, Stanford, California. “One of the challenges is having the availability of models that actually improve clinical care because we have some very early prospective trials on different devices, but we don’t have large-scale randomized clinical trials of AI devices showing definitive behaviors such as improved patient outcomes, that it helps curb skin cancer, or it catches it like dermatologists but helps reduce the biopsy load,” she said. “You need good data.”
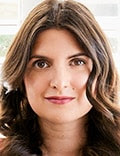
Roxana Daneshjou, MD, PhD
Another challenge she noted was overcoming biases built into medicine. “A lot of the image-based models are built on datasets depicting skin disease on white skin, and those models don’t work so well on people with brown and black skin, who have historically had worse outcomes and also have been underrepresented in dermatology,” said Daneshjou, an associate editor of NEJM AI.
There’s also the challenge of getting verified AI models into the clinic. “Similar to many medical AI endeavors, developing a proof-of-concept or research prototype is far easier and faster than bringing the development to real users,” Google’s Liu said. “In particular, it is important to conduct thorough validation studies on various patient populations and settings and understand how these AI tools can best fit into the workflow or patient journey.” A study last year documented progress Google made in deploying AI models in retina specialty clinics in India and Thailand, Liu noted.
Another challenge is to avoid overdiagnosis with these new technologies, Hartman at Brigham and Women’s Hospital said. Her group’s study showed the DermaSensor had a positive predictive value of 16% and a negative predictive value of 98.5%. “I think there’s some question about how this will factor into overdiagnosis,” she said. “Could this actually bombard dermatologists more if the positive predictive value’s only 16%?”
One key to dermatologists accepting AI tools is having a transparent process for validating them, Lee said. “Even with FDA clearance, we don’t have the transparency we need as clinicians, researchers, and advocates of machine learning and AI in healthcare,” she said.
But, Lee noted, the FDA in June took a step toward illuminating its validation process when it adopted guiding principles for transparency for machine learning-enabled devices. “Once we can get more access to this information and have more transparency, that’s where we can think about actually about making the decision to implement or not implement into local healthcare settings,” she said. The process was further enabled by a White House executive order last fall on the safe, secure, and trustworthy development and use of AI, she added.
The experience with telehealth during the COVID-19 pandemic, when patients and providers quickly embraced the technology to stay connected, serves as a potential template for AI, Lee noted. “As we’d seen with telehealth through the pandemic, you also need the cultural evolution and the development of the infrastructure around it to actually make sure this is a sustainable implementation and a scalable implementation in healthcare,” she said.
Lee had no relevant relationships to disclose. Hartman received funding from DermaSensor for a study. Witkowski is a cofounder of Sklip. Liu is an employee of Google Research. Daneshjou reported financial relationships with MD Algorithms, Revea, and L’Oreal.
Richard Mark Kirkner is a medical journalist based in the Philadelphia area.
Leave a Reply