The concepts were judged by reviewers and were not told who or what had created them.
By Gemma Conroy
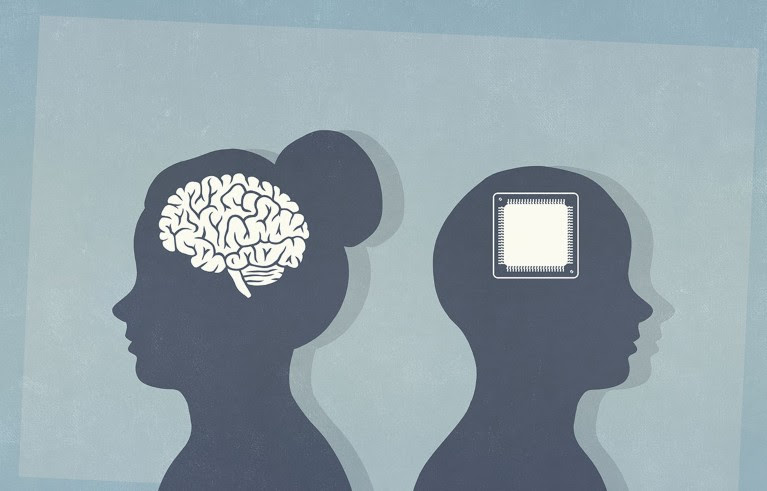
Researchers built an artificial intelligence tool that came up with 4000 novel research ideas in a matter of hours. Credit: Malte Mueller/Getty
An ideas generator powered by artificial intelligence (AI) came up with more original research ideas than did 50 scientists working independently, according to a preprint posted on arXiv this month1.
The human and AI-generated ideas were evaluated by reviewers, who were not told who or what had created each idea. The reviewers scored AI-generated concepts as more exciting than those written by humans, although the AI’s suggestions scored slightly lower on feasibility.
But scientists note the study, which has not been peer-reviewed, has limitations. It focused on one area of research and required human participants to come up with ideas on the fly, which probably hindered their ability to produce their best concepts.
AI in science
There are burgeoning efforts to explore how LLMs can be used to automate research tasks, including writing papers, generating code and searching literature. But it’s been difficult to assess whether these AI tools can generate fresh research angles at a level similar to that of humans. That’s because evaluating ideas is highly subjective and requires gathering researchers who have the expertise to assess them carefully, says study co-author, Chenglei Si. “The best way for us to contextualise such capabilities is to have a head-to-head comparison,” says Si, a computer scientist at Stanford University in California.
The year-long project is one of the biggest efforts to assess whether large language models (LLMs) — the technology underlying tools such as ChatGPT — can produce innovative research ideas, says Tom Hope, a computer scientist at the Allen Institute for AI in Jerusalem. “More work like this needs to be done,” he says.
The team recruited more than 100 researchers in natural language processing — a branch of computer science that focuses on communication between AI and humans. Forty-nine participants were tasked with developing and writing ideas, based on one of seven topics, within ten days. As an incentive, the researchers paid the participants US$300 for each idea, with a $1,000 bonus for the five top-scoring ideas.
Meanwhile, the researchers built an idea generator using Claude 3.5, an LLM developed by Anthropic in San Francisco, California. The researchers prompted their AI tool to find papers relevant to the seven research topics using Semantic Scholar, an AI-powered literature-search engine. On the basis of these papers, the researchers then prompted their AI agent to generate 4,000 ideas on each research topic and instructed it to rank the most original ones.
Human reviewers
Next, the researchers randomly assigned the human- and AI-generated ideas to 79 reviewers, who scored each idea on its novelty, excitement, feasibility and expected effectiveness. To ensure that the ideas’ creators remained unknown to the reviewers, the researchers used another LLM to edit both types of text to standardize the writing style and tone without changing the ideas themselves.
On average, the reviewers scored the AI-generated ideas as more original and exciting than those written by human participants. However, when the team took a closer look at the 4,000 LLM-produced ideas, they found only around 200 that were truly unique, suggesting that the AI became less original as it churned out ideas.
When Si surveyed the participants, most admitted that their submitted ideas were average compared with those they had produced in the past.
The results suggest that LLMs might be able to produce ideas that are slightly more original than those in the existing literature, says Cong Lu, a machine-learning researcher at the University of British Columbia in Vancouver, Canada. But whether they can beat the most groundbreaking human ideas is an open question.
Another limitation is that the study compared written ideas that had been edited by an LLM, which altered the language and length of the submissions, says Jevin West, a computational social scientist at the University of Washington in Seattle. Such changes could have subtly influenced how reviewers perceived novelty, he says. West adds that pitting researchers against an LLM that can generate thousands of ideas in hours might not make for a totally fair comparison. “You have to compare apples to apples,” he says.
Si and his colleagues are planning to compare AI-generated ideas with leading conference papers to gain a better understanding of how LLMs stack up against human creativity. “We are trying to push the community to think harder about how the future should look when AI can take on a more active role in the research process,” he says.
doi: https://doi.org/10.1038/d41586-024-03070-5
References
Si, C., Yang, D. & Hashimoto, T. Preprint at arXiv https://doi.org/10.48550/arXiv.2409.04109 (2024).
Leave a Reply