by Bob Yirka , Medical Xpress
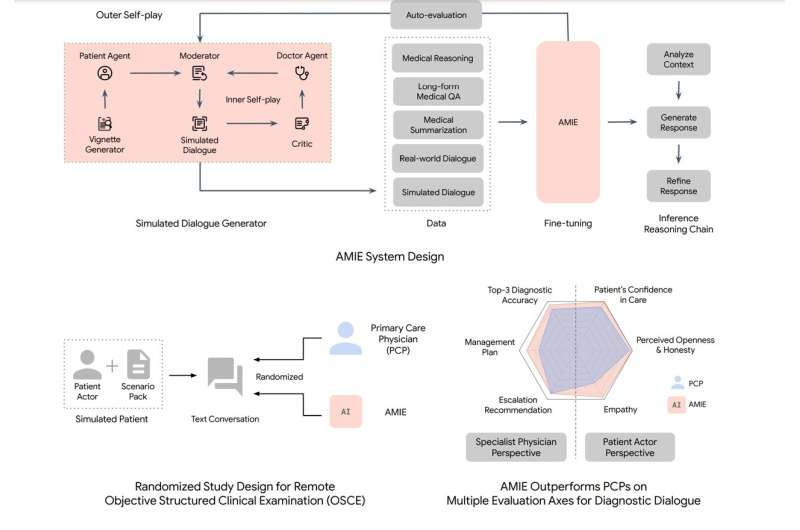
Overview of contributions. AMIE is a conversational medical AI optimized for diagnostic dialogue. AMIE is instruction fine-tuned with a combination of real-world and simulated medical dialogues, alongside a diverse set of medical reasoning, question self-play-based simulated dialogue environment with answering and summarization datasets. Notably, we designed a self-play based simulated dialogue environment with automated feedback mechanisms to scale AMIE’s capabilities across various medical contexts and specialities. Specifically, this iterative self-improvement process consisted of two self-play loops: (1) An “inner” self-play loop, where AMIE leveraged in-context critic feedback to refine its behavior on simulated conversations with an AI patient agent; (2) An “outer” self-play loop where the set of refined simulated dialogues was incorporated into subsequent fine-tuning iterations. During online inference, AMIE used a chain-of-reasoning strategy to progressively refine its response conditioned on the current conversation to arrive at an accurate and grounded reply to the patient in each dialogue turn. We designed and conducted a blinded remote Objective Structured Clinical Examination (OSCE) with validated simulated patient actors interacting with AMIE or Primary Care Physicians (PCPs) via a text interface. Across multiple axes corresponding to both specialist physician (28 out of 32) and patient actor (24 out of 26) perspectives, AMIE was rated as superior to PCPs while being non-inferior to the rest. Credit: arXiv (2024). DOI: 10.48550/arxiv.2401.05654
A team of AI researchers at Google Research and Google DeepMind has developed the rudiments of an AI-based diagnostic dialogue system to conduct medical interviews. The group has published a paper describing their research on the arXiv preprint server.
When a doctor interviews a patient to determine what medical situation needs to be addressed, the research team noted, they come armed with a deep background in medical education and practical experience. Though it might seem at times to a patient that such interviews are cursory at best, most are both efficient and result in accurate results.
But there is also room for improvement, the researchers note. One of the areas where many doctors fall short is in their bedside manner. Due to personality quirks, a tight and busy work schedule, or dealing with patients who are not always kind and polite, some medical interviewers may be seen as stiff or stilted, creating the perception that the doctor does not care much for the patient’s welfare.
In this new effort, the team at Google has noted that many LLMs, such as ChatGPT, often come across as quite empathetic and more than eager to help—traits sometimes lacking in doctor interviews. That gave them the impetus to begin working on an LLM that might one day evolve into a real-world diagnostic dialogue system.
The new system is called the Articulate Medical Intelligence Explorer, or AMIE. The team at Google is quick to point out that it is still purely experimental. They also note that building their system has been both unique and difficult due to the dearth of doctor/patient medical interviews available for use as training material.
That led them to try a novel approach to teaching their system how to query a patient. First, they trained it on the limited amount of publicly available data. They then attempted to coax the system into training itself by prompting it to play the part of a person with a specific illness. Next, they asked the system to play the part of a critic who has witnessed multiple interviews that the system conducted.
The system then interviewed 20 volunteers trained to pretend to be patients. The results were rated by medical professionals to determine accuracy. The volunteers who had played the patients evaluated the system’s bedside manner.
The researchers found AMIE to be as accurate in diagnosing the patients as trained doctors. They also found that it scored better with its bedside manner. The team at Google plans to improve the system’s capabilities when tested in more realistic real-word conditions and to improve its bedside manner further.
More information: Tao Tu et al, Towards Conversational Diagnostic AI, arXiv (2024). DOI: 10.48550/arxiv.2401.05654
Google Research blog: blog.research.google/2024/01/a … r-diagnostic_12.html
Journal information: arXiv
© 2024 Science X Network
Leave a Reply