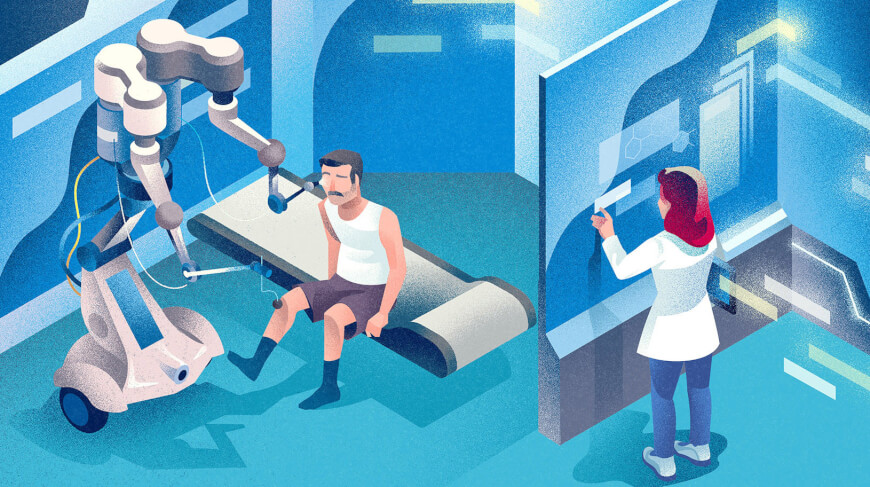
The potential of artificial intelligence for making healthcare better is indisputable. The question is how to integrate it successfully into our healthcare systems. For doing so, we have to overcome technical, medical limitations, as well as regulatory obstacles, soothe ethical concerns and mitigate the tendency to oversell the technology. The very first step should be to familiarize with what A.I. truly means. In our latest e-book, A Guide to Artificial Intelligence in Healthcare, we summarized everything you need to know, so now, let’s look at the second step: the challenges to overcome!
The transformative power of A.I. in healthcare
We have written extensively about the potential of artificial intelligence for redesigning healthcare. How it could help medical professionals in designing treatment plans and finding the best-suited methods for every patient. How it could assist repetitive, monotonous tasks, so physicians and nurses can concentrate on their actual jobs instead of e.g. fighting the tread-wheel of bureaucracy. By what means A.I. could prioritize e-mails in doctors’ inboxes or keep them up-to-date with the help of finding the latest and most relevant scientific studies in seconds. How its transformative power makes it as important as the stethoscope, the symbol of modern medicine, which appeared in the 19th century.
There are already great examples for its use in several hospitals: Google DeepMind launched a partnership with the UK’s National Health Service to improve the process of delivering care with digital solutions. In June 2017, DeepMind expanded its services – first of all, its data management app, Streams, to another UK hospital. IBM Watson is used at the Alder Hey Children’s Hospital as part of a science and technology facilities council project being run by the Hartree Centre. We asked the first experiences of physicians with the technology and gave an overview of the ever-expanding line of companies who are extensively investing in the development of the technology recognizing its transformative capability in healthcare.
However, the question we always have to face is how we translate the vast potential of artificial intelligence into everyday life. After the very first step – getting to know the most possible about A.I. in healthcare -, we should get a clearer picture of the obstacles.
Get the e-book here to read about the best companies, the way A.I. will redesign healthcare, the practical examples and ethical challenges!
1) Technological limitations of A.I.
The term “artificial intelligence” might be misleading in many cases as it implies a far more developed technology than it stands at the moment. At best, current technology – meaning various machine learning methods – is able to reach artificial narrow intelligence (ANI) in various fields. Yet, that is developing at an incredible speed. These narrowly intelligent programs defeat humans in specific tasks, such as IBM’s supercomputer Deep Blue winning at chess but unlike human world champions, these algorithms are not capable of also driving cars or creating art. Solving those other tasks requires other narrow programs to be built, and it is an immense challenge. Yet, there is incredible growth in computers’ ability to understand images and video – a field called computer vision – as well as text in the frames of natural language processing. The former is extensively utilized now in healthcare, for example in the field of medical imaging.
Michelle Zhou, who spent over a decade and a half at I.B.M. Research and IBM Watson Group before leaving to become a co-founder of Juji, a sentiment-analysis start-up, categorized ANI for The New Yorker here as recognition intelligence, and as the first stage of A.I. It means what algorithms running on ever more powerful computers can currently do is recognizing patterns and gleaning topics from blocks of text or deriving the meaning of whole documents from a few sentences. Yet, we are nowhere close to artificial general intelligence (AGI), that level of intelligence when a machine is capable of abstracting concepts from limited experience and transferring knowledge between domains.
2) Medical limitations
To avoid over-hyping the technology, the medical limitations of present-day ANI also have to be acknowledged. In the case of image recognition and using machine learning and deep learning algorithms for the purposes of radiology, there is the risk of feeding the computer not only with thousands of images but also underlying bias.
For example, the images tend to originate from one part of the U.S. or the framework for conceptualizing the algorithm itself incorporates the subjective assumptions of the working team. Moreover, the forecasting and predictive abilities of smart algorithms are anchored in previous cases – however, they might be useless in novel cases of drug side-effects or treatment resistance.
On the other hand, streamlining and standardizing medical records in such a way that algorithms can make sense of them mean another huge limitation in introducing ANI to hospital departments for doing administrative tasks. There are many hospitals where doctors still scribble their notes on patients’ files. How should the computer make sense of such notes if even the person who wrote that cannot read it two weeks later?
3) Ethical challenges
Yet, medical as well as technological limitations of A.I. as well as ANI will still be easier to overcome than ethical and legal issues. Who is to blame if a smart algorithm makes a mistake and does not spot a cancerous nodule on a lung X-ray? To whom could someone turn when A.I. comes up with a false prediction? Who will build in safety features so A.I. will not turn on humans? What will be the rules and regulations to decide on safety?
These complex ethical and legal questions should be answered if we want to reach the stage of AGI safely and securely. Moreover, ANI and at a certain point, AGI, should be implemented cautiously and gradually in order to give time and space for mapping the potential risks and downsides. Independent bioethical research groups, as well as medical watchdogs, should monitor the process closely. This is exactly what the Open AI Foundation does on a broader scale. It is a non-profit A.I. research company, discovering and enacting the path to safe artificial general intelligence. Their work is invaluable, as they are doing long-term research, and may help in setting up ethical standards on how to use A.I. on micro and macro levels. Perhaps also in the healthcare sector.
4) Better regulations
The FDA approved the first cloud-based deep learning algorithm for cardiac imaging developed by Arterys in 2017; which is a huge step towards the future. However, regulations around artificial intelligence generally lag behind or are literally non-existent. With the technology gaining ground and appearing in hospitals within the next 5-10 years, decision-makers and high-level policy-makers cannot allow themselves not to tackle the issue.
They should rather step ahead of the technological waves and guide the process of implementing A.I. in healthcare along the principles and ethical standards they work out with other industry stakeholders. Moreover, they should push companies towards putting affordable A.I. solutions on the table and keeping the focus on the patient all the time. Governments and policy-makers should also help in setting up standards on A.I. usage as we need specific guidelines starting from the smallest units (medical professionals) until the most complex ones (national-level healthcare systems).
5) Misconceptions and overhyping
Overhyping the capabilities of A.I. through marketing tactics and oversimplified media representations does not help but destroy a healthy image about how A.I. could contribute to healthcare. It also adds to the fog of confusion and misconceptions which need to be cleared up when we want to implement the technology successfully into our healthcare systems.
Definitions of machine learning, deep learning, smart algorithms, ANI, AGI or any other terms and concepts around A.I. need to be treated carefully. The same goes for its impact in healthcare. The story about Facebook shutting down an A.I. experiment because chatbots developed their own language and how they started conversing leaving out humans from the process was misrepresented by many news sites from India to Hong Kong, aggravating fears about A.I. becoming conscious and aiming for destroying the human race. And that’s just one example out of a swarm of similar articles.
6) Human rejection
Fears about A.I. eradicating humanity go hand-in-hand with exaggerated statements about A.I. coming for the jobs of medical professionals. Even Stephen Hawking said that the development of full artificial intelligence could spell the end of the human race. Elon Musk agreed. Moreover, artificial intelligence is said to take the jobs of radiologists, robots are surpassing the skills of surgeons, or aim to take many jobs in pharma. No wonder the medical community rejects A.I. Is it not enough for these smart algorithms to take over the world, are they also coming for our jobs?
The fears around A.I. are understandable as so few of us actually understand how the technology works down to the detail. And what we don’t understand, we tend to reject. Even more so, if thought-leaders or the media also tend to treat the issue with exaggeration and extremities. And although it will take time to get accustomed to the technology, we recommend everyone to be open-minded and familiarize with the concept of using A.I. in everyday life.
At the GPU Tech Conference in San Jose in May 2017, Curtis Langlotz, Professor of Radiology and Biomedical Informatics at Stanford University, compared the situation to that of the autopilot in aviation. The innovation did not replace real pilots, it augmented their tasks. On very long flights, it is handy to turn on the autopilot, but they are useless when you need rapid judgment. So, the combination of humans and machines is the winning solution. And it will be the same in healthcare. I agree with Langlotz completely when he says that artificial intelligence will not replace radiologists. Yet, those radiologists who use A.I. will replace the ones who don’t. Moreover, this enigmatic statement could also apply to ophthalmologists, neurologists, GPs, dentists, nurses or administrators. That’s why I reframed the above sentence to articulate the core message of The Medical Futurist team as succinct as possible.
Artificial Intelligence will not replace physicians. Yet, medical professionals who use A.I. will replace those who don’t.
Leave a Reply